INTRODUCTION
Chronic diseases and their complications are preventable with timely and effective care, disease prevention, and management strategies. Tobacco smoking is one of the leading modifiable risk factors contributing to chronic diseases and their complications1,2. Chronic tobacco use is a well-established major cause of endothelial cell damage in the blood vessels, which promotes plaque formation, inflammation, and development of chronic diseases such as cardiovascular diseases, stroke, chronic lung diseases, and cancer3. However, evidence suggests that smoking cessation can reverse endothelial damage, reduce inflammation, and significantly improve cardiovascular, lung, and gastrointestinal functions as early as 15 days post-cessation4-7. To combat this pressing public health issue in India, initiatives such as the National Tobacco Control Program (NTCP) and the National Program for Prevention and Control of Non-Communicable Diseases (NP-NCD) have been implemented to promote smoking cessation and reduce the burden of chronic diseases through the risk factor prevention approach8. These programs rely on pharmacological interventions, education, healthcare provider’s guidance, and self-determination, which shape individuals’ perception of the risks and benefits of smoking enabling smoking cessation9,10. However, many smokers in India underestimate the severe health consequences of smoking11.
Health behavior change, such as smoking cessation is often ‘triggered’ by significant events, such as the death of a loved one or the onset of a major diseases or self-determination12. For older adults, the diagnosis of chronic diseases, often coupled with functional decline, can serve as a critical warning, increasing self-awareness and motivating behavior change to improve health13. Behavior change is influenced by expectations about future health and survival, the perceived harm of quitting smoking, financial constraints due to disease onset, and frequent pressure to quit from healthcare professionals and family14. Many theories explain the process of behavioral changes among individuals where heightened ‘threat perception’, often triggered by a health diagnosis or risk awareness, serves as a cue to action, promoting preventive behaviors like smoking cessation. The Health Belief Model (HBM) offers a framework for understanding how individuals take action to prevent or manage illness based on perceived susceptibility, benefit, barrier, and self-efficacy15. The Theory of Planned Behavior further posits that behavior change is driven by the individual’s attitude toward smoking, others’ perception towards smoking, and the individual’s intent to control or quit smoking behavior16. For older adult long-term smokers, the onset of multiple health problems exacerbated by smoking can increase the ‘perceived threat’, motivating a shift towards healthier behavior13,15. For some patients, transitioning to a healthier lifestyle may become a necessity, which explains ‘cold turkey’ (unassisted quitting) among smokers with chronic diseases17.
In India, about 28.6% of Indian adults use tobacco, with roughly 100 million smokers18. Approximately one-fifth of older adults were diagnosed with at least one chronic disease19. While such diagnoses prompt individuals to reconsider their health behaviors, limited evidence exists on how these conditions influence smoking cessation among older adults in India. The study aims to identify the ‘perceived threat’ posed by chronic diseases as a ‘trigger’ for smoking behavior change among individuals aged ≥45 years, using nationally representative data. By identifying the factors that influence smoking cessation in this population, the study seeks to enhance our understanding of the drivers of behavior change in older adults, ultimately informing more effective public health interventions to reduce chronic disease risk.
METHODS
Data and sample
The current study utilized data from the first wave of the Longitudinal Ageing Study in India (LASI), conducted between April 2017 and December 2018, by the International Institute for Population Sciences, Harvard T.H. Chan School of Public Health, and the University of Southern California. LASI is a comprehensive, nationally representative survey focused on individuals aged ≥45 years in India. The primary objective of LASI was to assess the health, economic, psychological, and social well-being of older adults, providing insights into the lives of this demographic. LASI employed a multistage stratified area probability cluster sampling design to ensure the sample was representative nationwide. Data were collected from 72250 older adults aged ≥45 years, along with their partners, regardless of their age, covering all Indian states (except Sikkim) and Union Territories (UTs). The present study focuses specifically on a subset of this population, consisting of 66021 individuals aged ≥45 years, allowing an in-depth examination of the challenges older adults face in India.
Outcome variable
In this study, the main outcome of interest was smoking behavior among the older adults in India. In the LASI survey, the respondents were asked two sets of questions regarding smoking practices. The first question was ‘Have you ever smoked tobacco (cigarette, bidi, cigar, hookah, cheroot)?’ if the respondent answered ‘yes’, then they were asked: ‘Do you currently smoke any tobacco products (cigarettes, bidi, cigars, hookah, cheroot, etc.)?’. The responses were either ‘yes’ or ‘quit’. To assess smoking behavior, we categorize responses into three categories: ‘never smoked’, ‘quit’, and ‘currently smoking’. Quitting practices were assessed by asking three questions: ‘At what age did you completely stop smoking?’, ‘When were you first diagnosed with chronic conditions or diseases?’ and ‘How old were you at your last birthday?’. We constructed a new nominal variable, ‘quitting practices’, and subcategorized those who quit smoking into three categories: ‘quit before diagnosis’, ‘quit same year of diagnosis’, and ‘quit after diagnosis’. For those who currently smoked, the number of tobacco products consumed was measured by asking: ‘How many cigarettes, bidis, cigars, cheroot, etc., do you usually smoke in a day?’. Responses were categorized into three groups: ≤5, 6–10, and >10 cigarettes, bidis, cigars, or cheroot per day.
Explanatory variables
Health condition
A range of health measures, such as diagnosed morbidity, perceived health, and physical and mental impairment, were included as predictor variables in the analysis. Diagnosed morbidity was referred to as the presence of medical conditions in older adults. The LASI survey collected information on the self-reported prevalence of chronic diseases with the question: ‘Has any health professional ever diagnosed you with the following chronic conditions or diseases?’. Data on various groups of chronic morbidities were collected. A measure of diagnosed morbidity was generated by combining nine chronic conditions: 1) hypertension or blood pressure, 2) diabetes or high blood sugar, 3) cancer or a malignant tumor, 4) chronic lung disease, 5) chronic heart diseases, 6) stroke, 7) arthritis or rheumatism, osteoporosis or other bone/joint diseases, 9) neurological or psychiatric problems, and 9) high cholesterol. A categorical variable was created to indicate the presence of diagnosed morbidity: ‘0’ for no disease, ‘1–9’ for those who were diagnosed with only one of the listed chronic diseases, and ‘10’ for multi-morbidity, representing two or more diagnosed chronic conditions. Self-reported health was measured using a single question: ‘Overall, how is your health in general? Would you say it is: very good, good, fair, poor, or very poor?’. Those who responded ‘very good’ and ‘good’ were coded as 0-‘good’ health, those who reported ‘fair’ were considered as 1-‘fair’ health, whereas those who responded ‘very poor’ and ‘poor’ were coded as 2-‘poor’ health. Impairment was assessed with the question: ‘Do you have any form of physical or mental impairment?’ with response options of ‘yes’ or ‘no’.
Socio-economic and demographic characteristics
A set of socio-economic and demographic variables were considered and controlled in the analysis, including age (44–54, 55–64, and ≥65 years), sex (male, female), place of residence (rural, urban), education level (no schooling, primary, secondary, higher secondary or higher), currently married (yes, no), wealth quintile (poorest, poorer, middle, richer, and richest), employment status (never worked, worked at least 3 months during lifetime, currently working), and living arrangements (living alone, living with a spouse, living with others).
Statistical analysis
In this study, descriptive, bivariate, and multinomial logistic regression analysis were applied. Descriptive analysis (frequency and percentage) was used to describe the study sample. The bivariate analysis was conducted to assess the differentials in the pattern of smoking behaviors across all the explanatory variables. Chi-squared test was used to examine the unadjusted relationship between predictor variables and outcome variables. Multivariate analysis using multinomial logistic regression was used to investigate factors that best explain and predict smoking behaviors. Multinomial logistic regression analysis was used because the outcome variable had three categories (never smoked, quit, and currently smoking). The results of the regression analysis were presented as adjusted relative risk ratio (ARRR) along with a 95% confidence interval (CI). The multinomial logistic regression model made clear the factors associated with either quitting smoking or currently smoking using never smoked as reference category. Individual weight (IW) was used to account for the complex survey design and generalizability of the findings. All the analyses performed were done using Stata version 14.
RESULTS
Table 1 shows the percentage of smoking behaviors among individuals aged ≥45 years in India, categorized by health outcomes and various socio-economic and demographic characteristics. Overall, approximately 17% of individuals had ever smoked, of which 13% currently smoked, and 4% quit smoking. Smoking was particularly common among those diagnosed with stroke (23%), followed by lung disease (20%) and cancer (19%) than, individuals with hypertension (10%) and multi-morbidity (9%). Among smokers, no significant differences in smoking were observed based on perceived health status or physical and mental impairments. Smoking prevalence was slightly higher among those aged 55–64 years (15%) compared to other age groups. A significant gender difference existed, with around 26% of males currently smoking compared to only 3% of females. Smoking was more prevalent in rural areas (16%) than in urban areas (8%). Among education level categories, individuals with primary education had the highest percentage of current smokers at 16%. Additionally, married individuals were nearly twice as likely to smoke (15%) compared to their unmarried counterparts (8%). Smoking behavior did not vary substantially across wealth quintiles, with current smoking percentages ranging from 12.1% to 14.1%. However, smoking behavior differed notably by employment status; those who had worked at least 3 months during their lifetime were more likely to currently smoke (15%) than those who had never worked or were currently not working (8%). Living arrangements also influenced smoking behavior, with those living alone having the lowest percentage of current smokers (3.1%), while those living with others (19%) or with a spouse (14%) had higher percentages.
Table 1
Percentage of smoking behaviors among older adults aged ≥45 years in India, 2017–2018 (N=66021)
Background characteristics | Smoking behaviors | n | ||
---|---|---|---|---|
Never smoked | Quit | Currently smoke | ||
Total | 82.9 | 3.8 | 13.2 | 66021 |
Morbidity*** | ||||
No morbidity | 81.8 | 3.0 | 15.2 | 35136 |
Hypertension | 86.9 | 3.5 | 9.6 | 8642 |
Diabetes mellitus | 85.1 | 4.6 | 10.3 | 2250 |
Cancer | 76.3 | 5.2 | 18.5 | 187 |
Lung disease | 70.8 | 9.0 | 20.2 | 1490 |
Heart disease | 77.1 | 10.5 | 12.5 | 451 |
Stroke | 69.8 | 7.5 | 22.7 | 248 |
Arthritis | 83.2 | 3.4 | 13.4 | 4207 |
Neurological diseases | 79.4 | 5.9 | 14.6 | 469 |
High cholesterol | 84.3 | 3.0 | 12.7 | 298 |
Multi-morbidity | 85.6 | 5.3 | 9.1 | 12644 |
Self-rated health*** | ||||
Good | 83.6 | 3.0 | 13.4 | 27298 |
Fair | 83.6 | 3.4 | 13.0 | 26962 |
Poor | 80.2 | 6.1 | 13.7 | 11032 |
Impairment (physical/mental) *** | ||||
No | 83.2 | 3.5 | 13.3 | 61171 |
Yes | 80.3 | 7.1 | 12.6 | 4816 |
Age (years) *** | ||||
44–54 | 85.4 | 2.3 | 12.2 | 24301 |
55–64 | 81.8 | 3.4 | 14.7 | 20259 |
>65 | 81.3 | 5.7 | 13.0 | 21462 |
Sex*** | ||||
Male | 66.9 | 7.4 | 25.8 | 30710 |
Female | 96.5 | 0.9 | 2.7 | 35312 |
Place of residence*** | ||||
Rural | 80.4 | 4.1 | 15.5 | 42948 |
Urban | 88.5 | 3.3 | 8.2 | 23074 |
Education level*** | ||||
No schooling | 83.9 | 3.2 | 12.9 | 31130 |
Primary | 78.5 | 5.0 | 16.4 | 16208 |
Secondary | 81.9 | 4.2 | 13.9 | 12158 |
Higher secondary or higher | 89.6 | 3.7 | 6.8 | 6525 |
Currently married*** | ||||
Yes | 80.8 | 4.1 | 15.1 | 49492 |
No | 89.0 | 3.0 | 8.1 | 16528 |
Wealth quintile*** | ||||
Poorest | 83.8 | 3.6 | 12.6 | 13055 |
Poorer | 83.0 | 3.9 | 13.2 | 13298 |
Middle | 82.1 | 3.8 | 14.1 | 13260 |
Richer | 81.7 | 4.2 | 14.1 | 13302 |
Richest | 84.1 | 3.8 | 12.1 | 13107 |
Employment status*** | ||||
Never worked | 89.2 | 2.5 | 8.3 | 2316 |
Worked at least 3 months during life time | 80.7 | 4.2 | 15.1 | 48200 |
Currently working | 88.7 | 3.0 | 8.3 | 15506 |
Living arrangements*** | ||||
Living alone | 96.0 | 0.9 | 3.1 | 18435 |
Living with spouse | 79.3 | 6.7 | 14.0 | 17377 |
Living with others | 77.7 | 3.8 | 18.5 | 30153 |
Table 1 also presents quit rates among individuals according to health outcomes and socio-economic and demographic characteristics, revealing substantial differences across selected covariates. Quit rates were higher among those with heart disease (11%), followed by lung disease (9%), and stroke (8%). Individuals who rated their health as poor were more likely to quit smoking (6%) than those who rated their health as good (3%). Similarly, the quit rate was higher among those with physical or mental impairments (7%) compared to those without (4%). Quit rates increased with age, 6% of those aged ≥65 years having quit smoking, compared to lower percentages in younger age groups (2%). The quit rate was significantly higher among males (7%) than females (0.9%). Individuals living with a spouse were more likely to quit smoking (7%) than those living alone (0.9%).
The distribution of smoking quit rates based on disease profiles among older adults in India is presented in Figure 1. The quit rate before diagnosis was higher for all chronic diseases except cancer and stroke. For instance, among those diagnosed with chronic disease, individuals with high cholesterol had the highest quit rate before diagnosis (95%), followed by diabetes (73%), arthritis (69%), hypertension (65%), heart disease (53%), and lung disease (51%). In contrast, the quit rate after diagnosis was higher for cancer patients (68%), followed by those with neurological disorders (45%) and stroke (42%). The likelihood of quitting smoking in the same year of diagnosis was notably higher for stroke patients (17%), followed by those diagnosed with lung and heart diseases (15%).
Figure 1
Quitting practices of smoking according to disease profile among older adults aged ≥45 years in India, 2017-2018
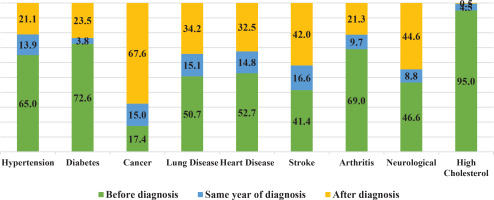
Figure 2 presents the distribution of the number of tobacco products smoked per day according to the disease profile of older adults in India. A notable observation was that nearly two-thirds of older adults diagnosed with cancer smoked more than ten cigarettes, bidis, cigars, cheroot etc. daily. There were also differences across disease profiles. For example, individuals with stroke (49%) and heart disease (35%) tend to smoke 6 to 10 tobacco products daily. However, a larger portion of individuals with high cholesterol (53%), neurological conditions (43%), arthritis (40%), and hypertension (39%) tended to smoke fewer (1–5) tobacco products per day.
Figure 2
Percentage of number of tobacco products (cigarettes, bidis, cigars, cheroot etc.) smoked in a day according to disease profile among older adults aged ≥45 years in India, 2017-2018
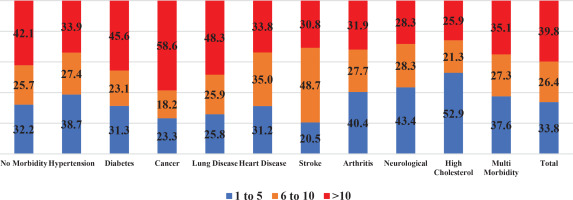
Table 2 presents the results of a multinomial logistic regression analysis examining the association between health outcomes (such as disease burden, perceived health, and physical and mental impairments), selected socio-economic and demographic characteristics, and smoking behaviors (quitting and current smoking). The adjusted relative risk ratio (ARRR) for quitting smoking was notably higher among individuals diagnosed with cancer (ARRR=2.68; 95% CI: 1.57–4.59), heart disease (ARRR=2.23; 95% CI: 1.63–3.05), and lung disease (ARRR=2.20; 95% CI: 1.82–2.67). Additionally, those with multi-morbidity (ARRR=1.20; 95% CI: 1.08–1.33) and hypertension (ARRR=1.18; 95% CI: 1.04–1.34) were more likely to quit smoking compared to individuals without any morbidity. Individuals who rated their health as poor (ARRR=1.72; 95% CI: 1.54–1.93) or fair (ARRR=1.17; 95% CI: 1.07–1.29) had a higher likelihood of quitting smoking than those who rated their health as good. Age also played a significant role, with individuals aged ≥65 years (ARRR=1.72; 95% CI: 1.54–1.92) and those aged 55–64 years (ARRR=1.28; 95% CI: 1.15–1.42) being more likely to quit smoking than those aged 45–54 years. The likelihood of quitting smoking was 89% lower among females compared to males (ARRR= 0.11; 95% CI: 0.10–0.12). Education was inversely associated with quitting smoking, as ARRRs were significantly lower among individuals with a higher education level compared to those with no schooling. Those who were not currently married were more likely to quit smoking (ARRR=1.41; 95% CI: 1.00–1.97) than those who were married.
Table 2
Multinomial logistic regression analysis of determinants of smoking behaviors among older adults aged ≥45 years in India, 2017–2018
Background characteristics | Quit | Currently smoke |
---|---|---|
ARRR (95% CI) | ARRR (95% CI) | |
Diagnosed morbidity (ref: no morbidity) | ||
Hypertension | 1.18 (1.04–1.34)*** | 0.89 (0.82–0.96)*** |
Diabetes mellitus | 0.99 (0.80–1.22) | 0.59 (0.51–0.69)*** |
Cancer | 2.68 (1.57–4.59)*** | 1.07 (0.66–1.72) |
Lung disease | 2.20 (1.82–2.67)*** | 1.18 (1.02–1.37)** |
Heart disease | 2.23 (1.63–3.05)*** | 0.80 (0.59–1.07) |
Stroke | 1.09 (0.64–1.87) | 1.10 (0.78–1.56) |
Arthritis | 1.00 (0.84–1.19) | 0.93 (0.84–1.03) |
Neurological disease | 1.08 (0.68–1.72) | 1.15 (0.87–1.51) |
High cholesterol | 1.91 (1.15–3.18)** | 0.90 (0.59–1.36) |
Multi-morbidity | 1.20 (1.08–1.33)*** | 0.68 (0.63–0.73)*** |
Self-rated health (ref: good) | ||
Fair | 1.17 (1.07–1.29)*** | 1.15 (1.08–1.21)*** |
Poor | 1.72 (1.54–1.93)*** | 1.43 (1.33–1.55)*** |
Impairment (physical/mental) (ref: no) | ||
Yes | 1.08 (0.95–1.24) | 0.86 (0.78–0.95)*** |
Age (years) (ref: 45–54) | ||
55–64 | 1.28 (1.15–1.42)*** | 1.15 (1.08–1.22)*** |
≥65 | 1.72 (1.54–1.92)*** | 0.95 (0.89–1.02) |
Sex (ref: male) | ||
Female | 0.11 (0.10–0.12)*** | 0.08 (0.08–0.09)*** |
Residence (ref: rural) | ||
Urban | 0.90 (0.82–0.98)** | 0.76 (0.72–0.81)*** |
Education level (ref: no schooling) | ||
Primary | 0.99 (0.90–1.10) | 0.78 (0.73–0.83)*** |
Secondary | 0.86 (0.77–0.96)*** | 0.53 (0.49–0.57)*** |
Higher secondary or higher | 0.61 (0.53–0.70)*** | 0.26 (0.23–0.29)*** |
Currently married (ref: yes) | ||
No | 1.41 (1.00–1.97)** | 1.38 (1.13–1.70)*** |
Wealth quintile (ref: poor) | ||
Poorer | 0.99 (0.87–1.13) | 1.14 (1.06–1.23)*** |
Middle | 1.14 (1.01–1.30)** | 1.30 (1.20–1.40)*** |
Richer | 1.40 (1.23–1.58)*** | 1.32 (1.22–1.43)*** |
Richest | 1.44 (1.27–1.64)*** | 1.31 (1.20–1.42)*** |
Employment status (ref: never worked) | ||
Worked at least 3 months during lifetime | 1.87 (1.25–2.78)*** | 1.43 (1.12–1.81)*** |
Currently working | 1.38 (1.05–1.80)** | 1.12 (0.95–1.32) |
Living arrangements (ref: live alone) | ||
Living with spouse | 2.66 (2.25–3.15)*** | 1.51 (1.37–1.67)*** |
Living with others | 1.90 (1.60–2.25)*** | 1.66 (1.51–1.83)*** |
ARRR: adjusted relative risk ratio. The variables adjusted in regression analysis for health condition (diagnosed morbidity, self-rated health, impairment) and selected socioeconomic and demographic characteristics (age, residence, education level, wealth quintile, employment status, and living arrangements).
Furthermore, individuals across all economic categories, except those in the poorer category, had a higher likelihood of quitting smoking than those in the poorest category. Employment status also influenced quitting behaviors, with individuals who had worked at least 3 months during their lifetime being 1.87 times more likely to quit smoking (ARRR=1.87; 95% CI: 1.25–2.78) compared to those who had never worked. Living arrangements were significantly associated with quitting smoking; individuals living with a spouse (ARRR=2.66; 95% CI: 2.25–3.15) or with others (ARRR=1.90; 95% CI: 1.60–2.25) were more likely to quit smoking than those living alone. Table 2 also explores the relationship between current smoking and various covariates, identifying significant predictors such as perceived health, age, marital status, household economic status, employment status, and living arrangements.
DISCUSSION
The study assessed the prevalence of smoking, smoking cessation, and the potential role of morbidity onset in influencing smoking cessation among older adults aged ≥45 years in India. Our analysis indicates that 13% of older adults currently smoke, and 4% have quit smoking. The findings highlight the complex interplay between health conditions, impairment status, living arrangement, and demographic factors in shaping smoking behaviors in this population. Quit rates were notably higher among individuals with cancer, heart disease and stroke. Specifically, quitting smoking after diagnosis was significantly higher among individuals with worrisome and severe diseases like cancer and stroke. This finding aligns with previous studies showing higher smoking cessation rates in the year of disease diagnosis for heart diseases, followed by stroke and cancer relative to years before the diagnosis of the respective diseases20 and a significant reduction of smoking prevalence among individuals diagnosed with these chronic diseases21. Heart disease, cancer, stroke, and neurological diseases like Alzheimer’s are the most feared diseases and are considered life-threatening and disabling, resulting in dependence on others and ultimately death22, suggesting that fear of life-threatening illness serves as a significant motivator for smoking cessation. Individuals diagnosed with severe diseases like cancer believe that smoking cessation improves treatment outcomes, prevents relapse, and mitigates risk, complications and the development of new diseases23. According to the Health Belief Model, individuals are more likely to take preventive actions, such as smoking cessation, when they perceive themselves at high risk for serious health consequences associated with smoking15.
As far as determinants are concerned, this study found a strong association between the onset of certain chronic diseases like cancer, heart disease and lung disease and higher likelihood of quitting smoking compared to those without any morbidity. These findings were consistent with previous research where it was found that individuals were more likely to be motivated to quit smoking upon diagnosis of cancer, heart disease, and stroke21. Interestingly, functional limitations due to physical and/or mental impairment did not influence quitting smoking. This result is supported by other studies that document that individuals with dementia or psychiatric illness were less likely to quit smoking24,25. Additionally, those who rated their health as poor or fair were significantly more likely to quit smoking compared to those who rated their health as good.
Our findings suggest that chronic diseases, poor self-rated health status, and various contextual factors such as age and living with a spouse may act as ‘threats’ that increase the perception of health risks. However, the mere onset of chronic diseases does not aid ‘threat perception’; rather, the interaction of multiple factors, including the temporal perception of health hazards, heightened risk perception and the intention to adopt preventive behaviors26. This heightened ‘threat perception’ plays a significant role in quitting smoking27. For instance, patients hospitalized with acute coronary syndrome (ACS or heart attack) often made serious attempts to quit smoking post-ACS episode, due to the pain and fear resulting from the episode28. This strong association of quitting smoking behavior among those with diagnosed chronic diseases offers scope for opportunistic counseling by healthcare providers emphasizing cessation behavior during this stage of heightened ‘threat perception’, effectively promoting quitting behavior. The time of diagnosis can be an effective moment for risk communication since patients may be receptive to cessation information23.
The influence of living arrangements and smoking cessation was highlighted in our multivariate analysis. Individuals living with a spouse were significantly more likely to quit smoking than those living alone, suggesting the potential benefit of involving a partner/spouse in the cessation program. In addition, healthcare professionals and family counseling interventions can play a critical role in cessation during the event of hospitalization and follow-up; and strong oversight and support by the family could be critical for the maintenance of smoking cessation thereafter28. However, patients may prefer to receive balanced information regarding both the risks of smoking and the benefits of cessation23, as this approach fosters trust in healthcare professionals. By highlighting the perceived harm of cigarette smoking, patients are able to recognize its negative impact on their health, which can ultimately motivate them to quit smoking.
Despite the apparent ‘threat perception’, this study also shows individuals diagnosed with chronic diseases continue to smoke; 56% to 80% of individuals diagnosed with chronic diseases smoked more than six cigarettes/bidis/cigars/cheroots etc. per day, possibly attributed to failure to fully perceive the harm and the intensity of cigarette smoking. Light smokers were more likely to quit smoking than intermittent and heavy smokers29 with diagnosed chronic conditions30, suggesting that the intensity of smoking and the individual’s perception of smoking-related harm are important factors influencing cessation behavior, stressing the need for patient-centric and diseases-specific counseling31.
According to the Transtheoretical Model, individuals with chronic diseases are more likely to contemplate and prepare to quit smoking when their ‘threat perception’ is heightened15. This study underscored that the period immediately following the diagnosis of chronic disease and subsequent follow-up, can serve as a ‘teachable moment’ for counselors and healthcare providers to promote smoking cessation13. This presents a crucial opportunity to integrate secondary prevention strategies at Tobacco Cessations Centers (TCC), Non-Communicable Diseases (NCD) Clinics and healthcare institutions. In India, TCCs operate at the district level, while NCD Clinics located within Community Health Centers (CHCs) are well-placed to function as the first point delivering tailormade, diseases-specific counselling closer to patients’ homes, especially in low-resource settings31. Additionally, the expansion of the NP-NCD program in 2023 to include conditions like chronic obstructive pulmonary disease (COPD) and asthma, chronic kidney disease (CKD), and non-alcoholic fatty liver disease (NAFLD) by the Government of India, provides a timely opportunity to incorporate cessation counselling at the stage of chronic diseases diagnosis at NCD clinics32. There is no question about the critical need to quit smoking as a primary preventive strategy; however, this study indicates that ‘threat perception’ immediately post-diagnosis of chronic diseases can be seized as a secondary prevention approach towards adult smoking cessation. A collaborative approach with family, health professionals, and counselors can significantly enhance the likelihood of successful tobacco cessation and its long-term maintenance, ultimately preventing further health complications/relapses and improving the overall health of older adults in India21,28.
Strengths and limitations
The strengths of this study are the large sample size, methodological rigor, and structured survey of nationally representative data of the population aged ≥45 years. However, there are some limitations to this study. Tobacco use, particularly cigarette smoking, is consistently under-reported in India, especially among women; hence, it may not provide comprehensive understanding considering gender differentials. Moreover, the data on morbidities were self-reported, missing a large number of undetected cases and the possibility of recall bias. Fourth, the data are from one wave, and therefore the longitudinal effects of chronic disease diagnosis over quitting behavior could not be studied.
CONCLUSIONS
Our findings suggest that the diagnosis of chronic diseases results in a heightened sense of ‘threat perception’ among individuals aged ≥45 years in India acting as a ‘trigger for change’ in smoking behavior. Additionally, smoking cessation behavior appears to be higher among those who consume fewer cigarettes per day, and those diagnosed with chronic diseases perceived as alarming by the community. Timely intervention by healthcare providers, family members, and counselors, aimed at identifying the ‘threat perception’ associated with the diagnosis of severe chronic diseases, coupled with subsequent follow-up care, can facilitate effective counseling and promote smoking cessation among older adults in India. This study contributes to the development of multilevel tobacco cessation policies and programs from a public health perspective, particularly within LMICs, considering the current epidemiological transition.